Table of contents
While the term artificial intelligence (AI) was introduced by Alan Turing in 1950, debates surrounding it have only recently taken center stage and are likely to persist fervently in the future. AI technology has rightfully assumed the status of a cornerstone in modern innovations, continuing to transform people's everyday lives: self-driving cars, facial and speech recognition devices, autonomous vacuum cleaners, mobile navigation apps, and other technologies all rely on AI to perform tasks.
However, delving deeper, many questions arise. What exactly is AI? How does it work? What types exist? What remarkable capabilities does it possess? And what are AI solutions? In this article, we aim to address these fundamental questions, providing insights that illuminate the essence of artificial intelligence.
Key AI Concepts
AI often comes with several essential terms like Machine Learning, Data Science, Generative AI, etc. Let's delve into these concepts and describe them.
Data science
In simple words, data science revolves around data, for example, statistics or data analytics. Businesses use data science to unveil actionable insights within their organization's data for informed decision-making and strategic planning.
This concept is closely connected with big data, referring to datasets that surpass one terabyte of data. However, on the organizational scale, terabytes of data have become commonplace for businesses, resulting in the term losing its popularity.
Artificial intelligence
At its core, AI refers to developing computer systems that aim to imitate human behavior and perform specific tasks that require human intelligence. It extends beyond traditional business problem-solving or B2C applications, encompassing robotics, hardware development, and even entertainment, such as gaming.
There are two categories of AI:
- General AI (Artificial General Intelligence) aims to replicate human-like cognitive abilities across various tasks.
- Narrow AI (Artificial Narrow Intelligence) is designed for specialized functions, excelling in specific domains.
Machine learning
Machine learning encompasses AI and data science capabilities. It is a set of algorithms that consume large amounts of data to predict, classify, or perform tasks closely aligned with human capabilities.
The application of ML is broad, and these are just a few examples:
- Computer vision uses ML to interpret visual data, applied in image recognition and object detection.
- Face ID is a machine learning-based facial recognition system that enhances security on devices like smartphones.
- Voice recognition uses ML to convert spoken language into text and is seen in virtual assistants and speech-to-text applications.
- Market demand forecasting uses ML techniques to analyze historical sales data to predict future demand, aiding businesses in optimizing inventory and supply chain, for example, in retail and E-commerce.
Generative AI
Generative AI lies at the intersection of data science, AI, and ML. To be more precise, it’s a highly complex machine learning model that has been trained on an extensive dataset to generate new data.
To gain a clearer understanding, let's introduce the term deep learning. Deep learning is a subfield of machine learning that focuses specifically on neural networks with many layers (deep neural networks). Generative AI is part of deep learning techniques, comprising colossal neural networks that have the ability to generate new, synthetic data that is similar to the training data they were exposed to.
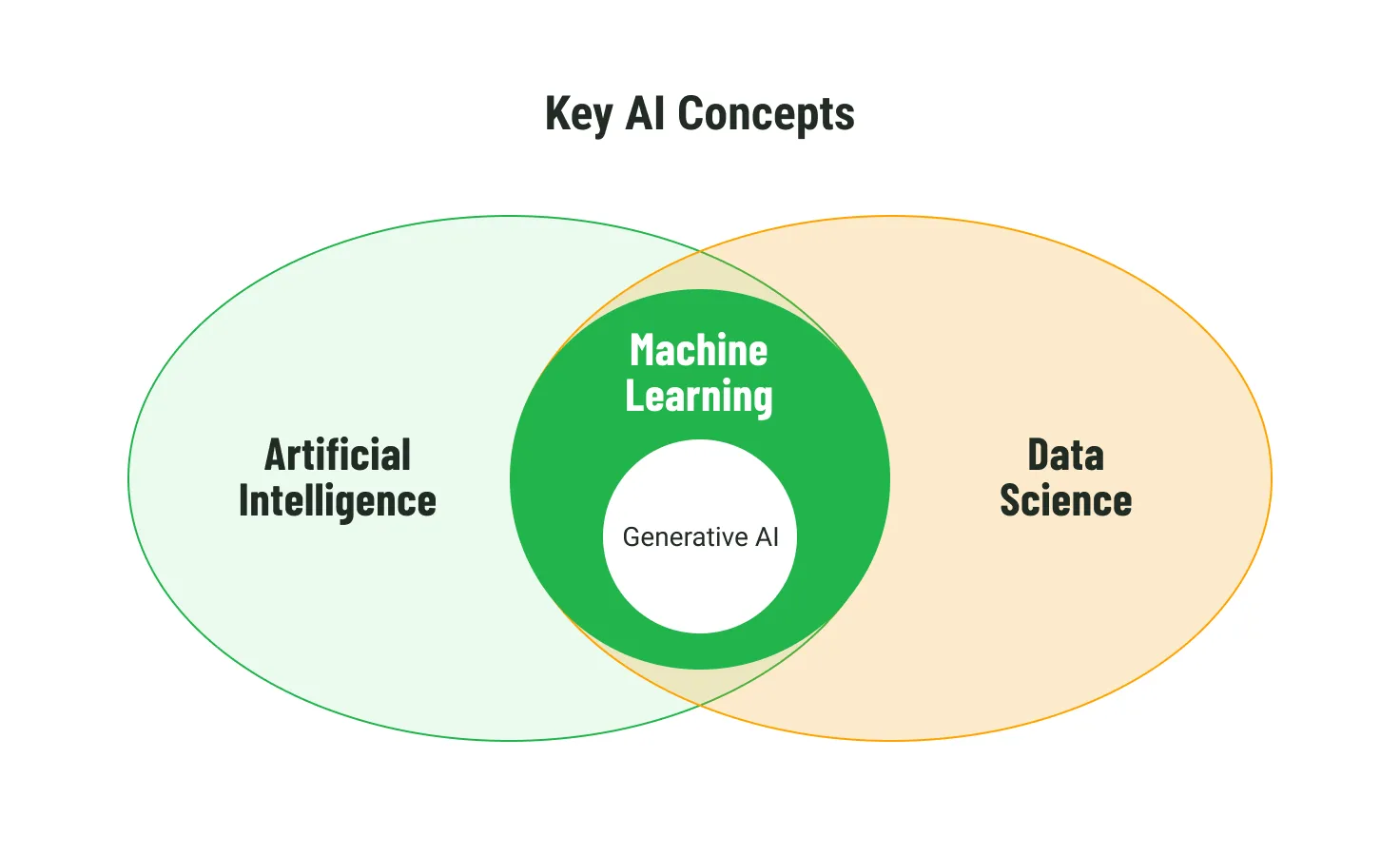
How Does ML Work?
When we talk about machine learning, a commonly used analogy is a black box. Within this metaphorical black box, there is a vast amount of data, forming the foundation for the model. Making the model perform specific tasks like detection of recognition requires supervised learning, where the model is trained by providing it with guidance.
Additionally, there is unsupervised learning that doesn't require human involvement in training but solely relies on data itself. However, it’s less popular compared to supervised learning.
Training an ML model
Imagine a dataset, such as the MNIST dataset, featuring pictures with hand-written numbers and corresponding labels. This is the training process stage, where the model learns from these pictures. The larger the dataset, the better the model performs. Once trained, the model solves similar tasks without human assistance. This process is called inference.
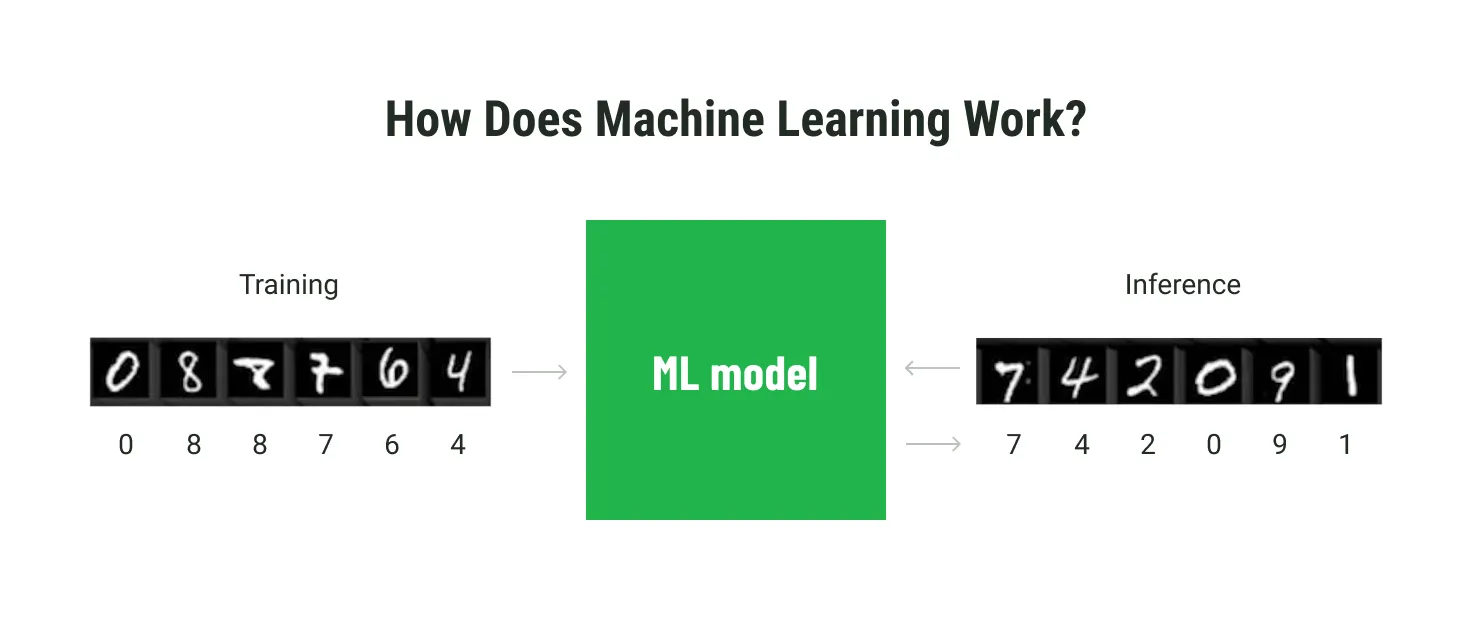
The process aligns with how humans train, for instance, teaching a child to recognize digits by showing images and assigning names to them. However, the model encounters challenges when faced with unfamiliar symbols during inference. While it may still provide an answer, that answer can be incorrect.
Here are some conditions for ML success:
Huge, high-quality, and relevant data
Training a model on a small dataset of 1,000 images from MNIST may result in an accuracy of around 70%. However, expanding the dataset to 50,000 images can significantly improve accuracy, exceeding 99%.
Alignment with past experiences
In our hand-written digit example, the model, trained on conventional digits, will fail when introduced to a new input like Japanese symbols that were not presented in the training data.
Solvable tasks
The model performs well when provided with tasks that can be resolved by a domain expert without relying on AI.
How Does Generative AI Work?
Generative AI operates on models that have undergone extensive training on large datasets, enabling them to comprehend prompts or instructions written in natural language. In contrast to traditional models designed for specific tasks, generative AI provides a more versatile solution. It can also be compared to a black box, but one containing a model with a human-like capability to generate diverse content.
Generative AI spans four primary directions: text, image, video, and audio. Text, particularly with models like GPT, gained significant popularity about a year ago, drawing attention to the broader field of generative AI.
Training Generative AI
Training generative AI requires substantial computational power, and that is one of the reasons why the technology has emerged just in recent years. The rise of GPU processor units was a game-changer. It eased up the hardware limitations we had, boosting both general information learning and the evolution of generative AI, opening the door to a new level of possibilities.
The price associated with training these models is a critical consideration. While official data on the cost of training from scratch remains unavailable, estimates indicate substantial figures, ranging from millions to over a hundred million dollars for models like GPT-4. This underscores the significant financial investment required to train such advanced models, potentially posing a barrier for startups with limited resources.
The Bottom Line
To sum up, Artificial Intelligence (AI) involves developing computer systems to imitate human behavior. Machine Learning, a subset of AI, uses AI algorithms to predict, classify, or perform tasks aligned with human capabilities. Generative AI is a complex ML model trained on extensive datasets, capable of generating synthetic data.
Whether you're exploring how AI can elevate your business or seeking to optimize existing implementations, we invite you to contact Emerline for tailored insights and solutions. Emerline has a team of 30 AI experts who deeply understand how AI works. Our impressive portfolio includes successful cases, particularly in mobile AI solutions development. We are ready to help your business maximize the potential of AI, ensuring that you get the most out of this transformative technology.
Update: January 8, 2024